Computational brain dynamics and function
Researchers from Stockholm University and KTH Computational Biology are collaborating with Swedish and European colleagues to produce better models of the human brain, which have many uses, such as early detection of brain-related illnesses.
by Anders Lansner, Jeanette Hellgren Kotaleski, Pawel Herman, Omar Gutierrez Arenas and Olivia Eriksson,
KTH Department of Computational Biology
Researchers from the Department of Computational Biology at the KTH Royal Institute of Technology and the Department of Numerical Analysis and Computer Science at Stockholm University are studying how our brains work. Anders Lansner, Jeanette Hellgren Kotaleski and Pawel Herman are working on modelling the neural systems of the brain to understand how it functions, both at a biologically detailed level and from a less detailed computational perspective. Along with postdoctoral researchers, Omar Gutierrez Arenas and Olivia Eriksson, and other colleagues and postgraduate students, they develop and simulate multi-scale and large-scale brain networks in an attempt to integrate the huge amounts of available biological data into coherent mathematical models that give insights into the mechanisms underlying the brain’s impressive information processing capabilities.
This research is being conducted in close collaboration with experimental neuroscientists at the Karolinska Institute in Stockholm and with European researchers via EU projects (such as the Human Brain Project) and together with the Swedish e-Science Research Centre (SeRC). The brain systems that are being studied include the cortex (the largest part of the brain), the hippocampus (a small part of the cortex that is important for learning and memory) and the basal ganglia (which is critical for decision-making and is primarily affected in conditions like Parkinson’s disease).
Biologically detailed models of the brain each address different levels of brain systems, such as the cascades of biochemical signals within individual brain cells that are triggered by neurotransmitters, or the electrical properties of individual neurons in the brain, or the networks of neurons that form brain microcircuits and connections between areas of the brain. These models vary in the amount of detail that they contain and in the extent to which they take into account information about how other levels of the brain work.
To make a more comprehensive model of the brain that builds on biological data at different levels of brain organization, the researchers are using the multi-scale computational approach. This will help to bridge the gaps in our understanding of how human behaviour and brain phenomena observed with the use of popular neuroimaging techniques such as functional magnetic imaging (fMRI) depend on the cascades of electrical signals between the brain cells in normal healthy brains.
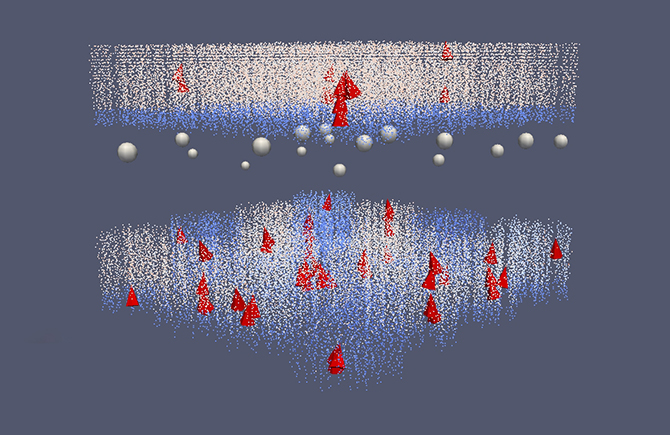
The researchers have recently modelled the cascade of biochemical signals inside a cell that is triggered by the activation of receptors involved in reward-dependent learning in part of the basal ganglia. Modelling such systems is necessary for understanding the mechanisms involved in memory and learning in the brain. However this is very complex: multiple sources of information are required when building models of this kind of subcellular signalling. To simulate such systems is computationally intensive and requires the use of high-speed supercomputers at PDC and elsewhere.
So far we have considered using computers to model brains, but it is also possible to look at how the brain processes information and to use that to develop new computing technologies. This leads us to another type of brain simulation that the researchers have been running on the supercomputer facilities at PDC. The long-term goal of this work is to identify the most significant aspects of the detailed brain models and to incorporate them into a framework based on more abstract brain theories. This could then be used to produce a system that can analyse large amounts of complex data in the same way that the brain processes multiple streams of sensory information. To date the researchers have run some of these types of simulations on the Cray supercomputers at PDC. As a result they have developed a “cortex-inspired” computational framework for performing advanced analysis of brain imaging recordings from various types of medical scanning, such as fMRI and positron emission tomography (PET).
To diagnose and cure human brain diseases, we need to be able to identify abnormal patterns in the ongoing brain activity that correspond to the actual illness. The vast amounts of data that are being generated by modern medical scanning equipment are too large for clinicians to analyse by eye and too complex to be assessed with the current conventional methods. Thus society at large has a problem where vital information (which might, for example, lead to being able to detect and diagnose illnesses early enough to effect a cure before the problem becomes serious) is not being fully utilised.
To address this problem, the KTH researchers are currently working towards enhancing their existing analysis method to make it possible to identify and study relevant patterns in electroencephalography (EEG) and magnetoencephalography (MEG) neuroimaging data. They want to be able to identify patterns both in spatial terms (relating to different areas in the brain) and in temporal terms (looking at what happens over time in specific parts of the brain). At present they are focusing on developing the large-scale computational framework to adequately model the brain’s information processing machinery and to analyse neuroimaging data – the main diagnostic aspects of the work are yet to come.
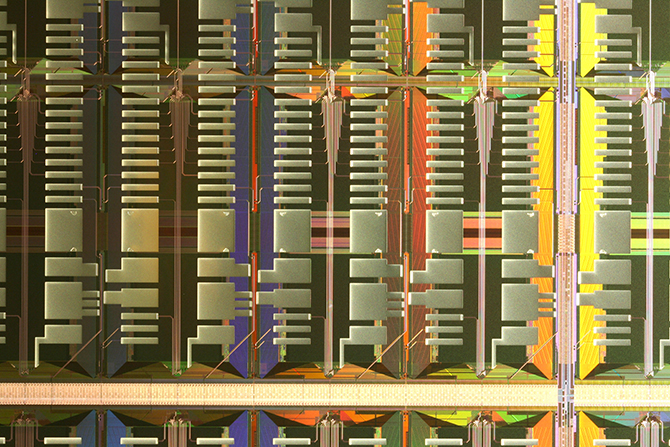
To effectively handle such vast quantities of data, the researchers’ neural-network-based brain models need to be very large and hence require so many calculations that the simulations have to be run on very fast supercomputers. However, the configurations of different supercomputer systems and their components, along with the way the code takes advantage of the parallel processing in each supercomputer, mean that there can be variations in how fast and how efficiently code runs on different systems. Therefore the researchers are also investigating ways to implement their simplified large-scale brain network models efficiently on novel types of massively parallel hardware like the SpiNNaker system (a computer architecture specifically designed to simulate the human brain) and the Epiphany chip from Adapteva, as well as on a custom-designed very-large-scale integration (VLSI) chip, which is being developed in collaboration with the KTH School of Information and Communication Technology.
Note:
- Anders Lansner is affiliated with both the KTH Department of Computational Biology and the Department of Numerical Analysis and Computer Science, Stockholm University.
- Jeanette Hellgren Kotaleski and Omar Gutierrez Arenas are affiliated with both the KTH Department of Computational Biology and the Science for Life Laboratory, School of Computer Science and Communication, KTH.
- Olivia Eriksson is affiliated with both the KTH Department of Computational Biology and the Science for Life Laboratory, Department of Numerical Analysis and Computer Science, Stockholm University.